**How Can Artificial Intelligence Help to Reduce Appraisal Bias in the Future?**
Performance appraisals are a critical component of workplace dynamics, influencing career progression, compensation, and employee satisfaction. However, traditional appraisal processes are often fraught with biases—both conscious and unconscious—that can lead to unfair evaluations and inequitable outcomes. As organizations strive to create fairer and more inclusive workplaces, artificial intelligence (AI) is emerging as a powerful tool to mitigate appraisal bias. By leveraging advanced algorithms and data-driven insights, AI has the potential to transform how employee performance is assessed, making the process more transparent, objective, and equitable. But how can AI truly address such a deeply rooted issue?
To explore this question, it’s essential to examine the strategies and practices that can enable AI systems to effectively reduce bias in appraisals. First, ethical AI development and bias mitigation techniques must be prioritized to ensure that AI systems are designed with fairness in mind from the outset. Next, organizations must implement transparent and explainable AI models to build trust and allow stakeholders to understand how decisions are made. Another critical factor is the use of diverse and representative training data to prevent AI systems from perpetuating existing biases. Furthermore, continuous monitoring and auditing of AI systems can help identify and address emerging issues over time. Finally, fostering collaboration between AI developers and human resource professionals can bridge the gap between technological capabilities and workplace realities, ensuring that AI tools align with organizational goals and values.
In this article, we will delve into these five subtopics to provide a comprehensive overview of how AI can drive meaningful change in reducing appraisal bias. By embracing these strategies, organizations can harness AI not only as a technological innovation but also as a catalyst for creating fairer, more inclusive workplaces in the future.
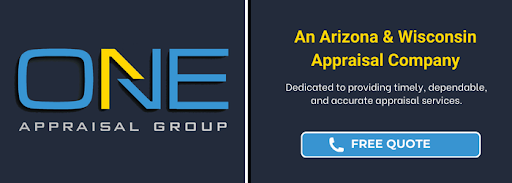
Ethical AI Development and Bias Mitigation Techniques
Ethical AI development and bias mitigation techniques are pivotal in addressing appraisal bias in the future. Bias in appraisal processes, whether in hiring, performance evaluations, or promotions, can perpetuate inequality and hinder diversity within organizations. Artificial intelligence, when developed and deployed ethically, has the potential to reduce these biases by introducing more objective, data-driven decision-making systems. However, achieving this requires developers and organizations to prioritize ethical considerations and actively implement strategies to mitigate bias in AI systems.
One of the primary approaches to ethical AI development is identifying and addressing bias during the design phase. This involves conducting thorough assessments of the algorithms and datasets used to ensure they do not reflect or amplify societal prejudices. For instance, if an AI system is trained on historical appraisal data that contains inherent gender or racial biases, the system may replicate and even exacerbate these biases. By employing techniques such as fairness-aware machine learning and bias detection algorithms, developers can pinpoint and correct these issues before deployment.
Additionally, ethical AI development emphasizes the importance of accountability and governance in the creation of AI systems. Organizations must establish clear guidelines and principles for ethical AI use, such as fairness, transparency, and inclusivity. These guidelines should be enforced through interdisciplinary collaboration, with input from ethicists, sociologists, and industry experts, alongside AI developers. By fostering a culture of accountability, companies can ensure their AI systems are designed with a commitment to equity and impartiality, reducing the risk of biased appraisals.
Overall, ethical AI development and bias mitigation techniques offer a roadmap for leveraging AI to create fairer and more equitable appraisal systems. By prioritizing ethics and proactively addressing bias, organizations can harness the power of AI to promote inclusivity and objectivity in decision-making processes, ultimately fostering a more just and equitable workplace.
Implementation of Transparent and Explainable AI Models
Implementing transparent and explainable AI models is a crucial step in reducing appraisal bias in the future. Traditional AI systems often operate as “black boxes,” making decisions without providing insight into the reasoning behind them. This opacity can perpetuate or even exacerbate biases embedded in the data or algorithms, as there is no clear way to identify or address problematic patterns. Transparent and explainable AI, on the other hand, provides clarity about how decisions are made, making it easier to detect and correct biases in the system.
One of the key advantages of explainable AI is its ability to foster trust between human users and AI systems. When individuals understand the logic behind AI-driven decisions, they are more likely to accept and rely on these systems for critical tasks, such as employee performance appraisals. This transparency also empowers organizations to hold AI accountable, as decisions can be audited and challenged if necessary. For example, if an AI model disproportionately rates certain demographic groups lower during appraisals, explainability tools can help identify the root cause, whether it stems from biased data, flawed algorithms, or other factors.
Moreover, explainable AI models encourage ethical decision-making by providing a framework for fairness and accountability. By revealing how decisions are reached, stakeholders can ensure that the system aligns with organizational values and legal standards, such as non-discrimination policies. This is especially important in fields like human resources, where appraisal processes significantly impact employees’ careers. Transparency allows organizations to proactively address inequities and implement corrective measures before they lead to larger systemic issues.
In summary, transparent and explainable AI models are an essential component of reducing appraisal bias in the future. They promote accountability, trust, and fairness by shedding light on the decision-making process and enabling organizations to identify and rectify biases. As AI continues to play a larger role in workplace evaluations, prioritizing transparency will be key to creating equitable and effective appraisal systems.
Leveraging Diverse and Representative Training Data
Appraisal bias often stems from inherent gaps in the data being utilized to make decisions. In the context of artificial intelligence (AI), biased or incomplete datasets can perpetuate and even amplify existing prejudices, leading to unfair outcomes. Leveraging diverse and representative training data is a critical step in reducing these biases and ensuring that AI systems make equitable and accurate assessments.
When training AI models, the data used serves as the foundation for their decision-making capabilities. If the data lacks diversity and fails to represent a wide range of demographics, experiences, and perspectives, the AI may inadvertently favor certain groups while disadvantaging others. For example, an AI system designed to assist in employee appraisals might disproportionately favor candidates from well-represented groups if the training data primarily consists of individuals from those groups. Introducing diverse and representative training data ensures that AI systems are exposed to a broader array of scenarios and contexts, reducing the likelihood of biased predictions.
To achieve this, organizations must prioritize collecting and curating datasets that include inputs from various genders, ethnicities, cultural backgrounds, and socioeconomic statuses. Additionally, historical data that contains biased patterns should be carefully analyzed and adjusted to prevent the perpetuation of unfair practices. By taking these steps, AI systems can better reflect the diversity of the real world, leading to fairer outcomes in appraisals and evaluations.
Moreover, leveraging diverse training data goes beyond fairness; it enhances the overall performance and robustness of AI models. Systems trained on heterogeneous datasets are better equipped to handle complex, real-world scenarios and make more generalized decisions. This approach not only reduces bias but also builds trust in AI technologies as tools for fostering inclusivity and equity in professional environments.
“`html
Continuous Monitoring and Auditing of AI Systems
“`
Continuous monitoring and auditing of AI systems is a crucial step in reducing appraisal bias in the use of artificial intelligence. Bias in AI systems can often emerge or evolve over time, even if the initial design and training were carefully controlled. Regular monitoring ensures that the system’s performance aligns with ethical and fairness goals throughout its lifecycle. This process involves tracking how the AI behaves in real-world scenarios, identifying deviations from expected outcomes, and addressing any bias that arises promptly.
Auditing AI systems involves a systematic review of their decision-making processes to ensure they remain transparent and fair. This includes evaluating how the AI interprets data, the weighting of different variables, and whether any unintended biases influence its conclusions. By conducting regular audits, organizations can detect and correct issues before they lead to systemic discrimination or unfair outcomes. This practice not only helps maintain trust in AI-driven systems but also ensures compliance with legal and ethical standards.
Continuous monitoring and auditing also encourage accountability. When organizations commit to ongoing oversight, they demonstrate a willingness to take responsibility for their AI systems’ actions. This can foster public confidence and reassure users that the AI is being used responsibly. Furthermore, these practices can uncover broader systemic issues in data collection or organizational processes, leading to improvements that extend beyond the AI system itself. In the future, adopting robust monitoring and auditing frameworks will be indispensable for creating AI systems that are fair, transparent, and equitable.
Collaboration Between AI Developers and Human Resource Professionals
Collaboration between AI developers and human resource (HR) professionals is a crucial step toward reducing appraisal bias in the future. By fostering close partnerships, these two groups can combine their expertise to create AI systems that are not only technically robust but also aligned with the nuanced human factors that influence fairness and equity in performance evaluations. This collaboration ensures that AI tools are designed with a deep understanding of workplace dynamics, organizational goals, and the ethical considerations involved in appraisals.
HR professionals bring valuable insights into the specific challenges and biases that can occur during performance evaluations. These may include unconscious biases related to gender, race, age, or other factors, as well as systemic inequities in how performance metrics are applied. By working with AI developers, HR professionals can help identify the key areas where bias might arise and provide guidance on how to address them. For example, they can ensure that the algorithms account for context-specific nuances, such as differing job roles or team dynamics, which might affect appraisal outcomes.
On the other hand, AI developers contribute technical expertise in building systems that can process large amounts of data, identify patterns, and flag potential biases. By collaborating with HR professionals, they can ensure that their models are not only accurate but also interpretable and fair. This partnership enables developers to build tools that incorporate ethical AI principles, such as fairness, accountability, and transparency, while tailoring them to the real-world needs of organizations.
Moreover, this collaboration can extend to the development of training programs for HR teams to help them understand the AI tools they are using. By demystifying how these systems work, HR professionals can use AI more effectively and confidently, ensuring that the tools are being applied in a way that supports fair and equitable decision-making. Together, AI developers and HR professionals can create a feedback loop to refine and improve AI systems over time, making them even more reliable in reducing appraisal bias.